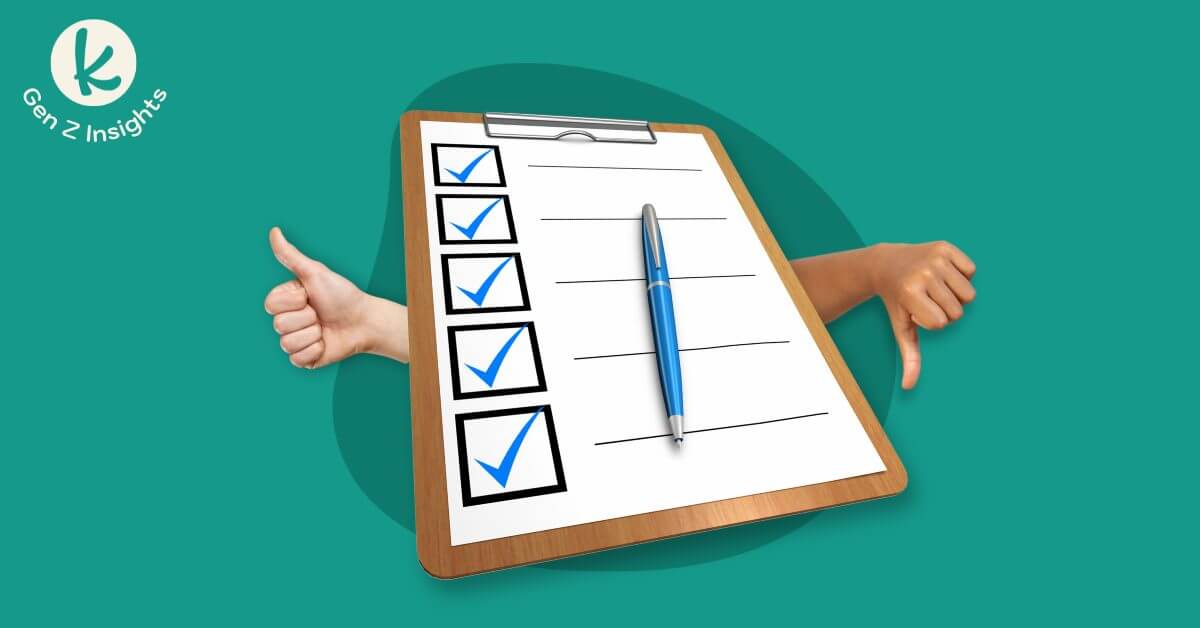
How to identify — and avoid — survey bias in market research
Put simply, survey bias describes a wide range of weaknesses that can creep into surveys, making them less accurate. People are not perfect, and neither are surveys. In fact, survey bias cannot be avoided completely, unless you start surveying robots who were all manufactured at the same plant with identical coding. If you’re sticking with humans — and we’re betting you are — the goal is to limit and acknowledge survey biases like these as much as possible:
Sampling Bias
Surveys are all based around extrapolating data off of samples because taking the time to interview every human alive isn’t possible. According to the UN, as of Jan 2022 there are an estimated 7.9 billion people on Earth. That’s a lot of data. So no survey interviews everyone. But how do organizations choose their survey sample? You could stand in Times Square and ask people who randomly walk by to participate in your survey. Your survey isn’t actually random, since you’re limited to people in Times Square in that time period who are able to walk and who agree to talk to you. What about the people who ignored you, were driving, or weren’t there at all? That’s sampling bias. Within sampling bias, you can run into a host of other biases, like:
Representation bias — when none of these things are not like the others
Representation bias refers to a survey that doesn’t accurately reflect the population you’re surveying. Let’s say you ask Gen-Z to take a survey on their phones, but your panel provider only optimizes the survey for the iPhone, every Android user is instantly excluded from the group and the data is flawed. In general, increasing sample size and making certain the survey is accessible to different groups within the population helps counter this.
Survivorship bias — unnatural selection
In survivorship bias, follow-ups are only taken with survey participants who want to keep participating, and often have an already favorable view of a company or topic. Pollfish has this great advice: “It is important to get feedback not just from your current customers–who are likely satisfied–and potential customers–who don’t know how they feel about you–but from your former customers who you likely failed to satisfy.” In other words, don’t solely follow-up with those who like your product, but those who don’t like it.
Exclusion bias — when you never go back to the well
Exclusion bias in definition terms: “Collective term covering the various potential biases that can result from the post-randomization exclusion of patients from a trial and subsequent analyses.” But let’s break that down in a way you can actually understand.
Exclusion Bias (or Attrition Bias) refers to removing previous participants from future surveys, and can skew results. For example if you initially surveyed people in San Francisco, then did a follow up survey a year later, but only surveyed people still living in San Francisco, you’ve chosen to exclude all participants who moved. This is easily avoided by keeping tabs of previous participants and their trends. This is the inverse of survivorship bias.
Response and questionnaire bias
Another major source of bias comes from the survey itself. Just as the data sample can be flawed, the survey has its own biases. Response bias comes in many forms. If your survey is in English, like this blog, your sample is limited to readers of English. Yet beyond the obvious, the real insidious biases sneak in through far more subtle ways. The most common biases occur from the order of the questions and the way they’re presented. Just as with sampling bias, there are specific biases that fall under the umbrella of response — or questionnaire bias:
Order bias — because everyone likes first place
How many courtroom dramas feature a judge banging their gavel, demanding the prosecutor to stop leading the witness? Yet, leading is exactly what many questions do by design. Order bias comes in two forms: the order of choices for each question, and the order of questions in the overall survey. Imagine the first question on a survey asks a respondent to rate their favorite meal of the day between breakfast, lunch, or dinner. Then, later on a respondent is asked how many meals they consume a day. They’re led to answer three, simply through the order those questions were presented, no matter how they define a meal or how many times they actually eat. Similarly, asking someone to rank dinner, lunch, or breakfast reads differently than asking someone to rank breakfast, lunch, or dinner. And for the record, breakfast is best. Of course, now that I’ve told you that you are biased in favor of breakfast.
What can you do to offset this bias? According to leadquizzes: “Reduce the number of scale-type questions and make your questions as engaging as possible, randomize your questions and answer options, group your questions around a common topic, and so on.”
Acquiescence bias — when getting a ‘yes’ gets you nowhere
Another survey bias is acquiescence bias, where respondents become accustomed to saying yes and agreeing with everything. In many surveys, participants end up with directly contradictory or flawed data sets. Often this type of response comes from either boredom with the survey, or a desire to please. By offering video-based responses, Knit excels at going deeper into participants’ feelings and opinions, and can avoid this type of bias.
Self-selection bias — or, ‘The Yelp high council will hear of this’
Self-selection bias is another common survey issue. In a volunteer survey, the survey is limited to volunteers who want to take it. This is one of the hardest biases to avoid. Even so-called mandatory surveys such as the US Census are limited by people’s willingness to participate. If a city mails a survey to residents asking if they should change an intersection, only those who actually care about the issue will respond. Many who do not mind the intersection as it is will not answer at all, skewing the results. In a similar vein, look up restaurant reviews and you’ll find only glowing and glaring. Those who had amazing experiences shared them, and those who had nightmares shared. But the vast majority of people don’t review at all. The key to fighting self-selection bias is randomization. The greater the randomization of the sample, the less bias will appear.
Extreme response bias — when there’s no traffic in the middle of the road
Another common bias is for survey participants to answer in the extreme even if they don’t hold extreme views. Extreme response bias often comes from the survey design, especially if worded using scales, and when similar types of questions are repeated. According to Pollfish, many participants start answering in the extremes when faced with repetition or with questions based on scales. This is another opportunity for nuance through video or other qualitative methodologies, or for the survey to include different types of questions that counter this bias and dig into a participant’s actual response.
Surveys are biased. It’s impossible for them to be otherwise. However, an awareness of survey biases and how to counter them creates cleaner and more reliable data. Thinking critically about design and fielding are one way to do that.
How video insights can combat survey bias
Another is reintroducing nuance through the use of video response tools and analysis. Video responses, whether they’re voice of consumer videos or shot as show-and-tells address common response and questionnaire biases by allowing analysts to observe the answering process.
When it comes to qualitative research, consumers don’t have to rely on memory to tell you what’s in their pantry, glovebox, or medicine cabinet — they can show you. Voice of consumer videos allow for respondents to tap into the full range of possible answers to a question, express sentiment in real time, and helps to remove the temptation to coast through your survey.
At Knit, we work hard to deliver the cleanest possible data to you with the least amount of survey bias possible when we scope, design, and program your study. We also go a step further: we work to deliver quant and qual video analysis in half the usual time. All so you can turn rich data analysis into actionable strategy.

Subscribe for industry insights delivered weekly.
Get all the Knit News you need with access to our free newsletter to stay a step ahead on the latest trends driving the industries of tomorrow.